Importance of Data in Training Customer Service Chatbots
Chatbots are becoming an increasingly popular tool for providing customer service in various industries. However, for a chatbot to effectively assist and engage with customers, it needs to be trained with relevant and high-quality data. The importance of data in training customer service chatbots cannot be overstated.
Data serves as the foundation upon which chatbots are built. It provides the necessary information and knowledge that the chatbot can use to understand and respond to customer queries. The more diverse and comprehensive the data, the better equipped the chatbot will be to handle a wide range of customer interactions. Data helps the chatbot learn about different customer preferences, common queries, and appropriate responses, enabling it to deliver accurate and relevant information in real-time.
In addition to enabling the chatbot to understand and respond to customer queries, data also plays a crucial role in improving the chatbot over time. By continuously analyzing and learning from the data, chatbots can identify patterns, trends, and areas for improvement. This iterative process allows the chatbot to enhance its performance, becoming more efficient and effective in providing customer support. Ultimately, the success of a customer service chatbot heavily relies on the quality and relevance of the data used to train it.
Understanding the Role of Datasets in Developing Effective Chatbots
Developing effective chatbots requires a robust understanding of the role that datasets play in their development. Datasets serve as the foundation upon which chatbots are trained and equipped to interact intelligently with users. These datasets contain vast amounts of relevant customer service information, ranging from frequently asked questions to specific product information.
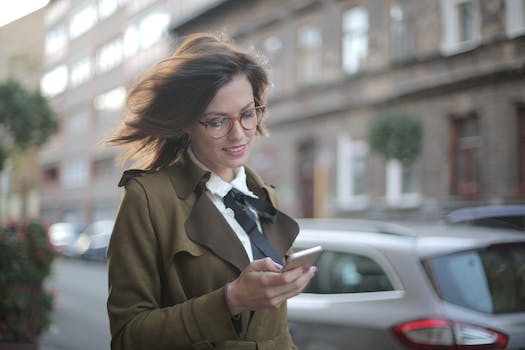
The role of datasets in chatbot development goes beyond just the information they contain. These datasets enable the chatbot’s machine learning algorithms to make connections between user queries and appropriate responses. Through the process of training, the chatbot learns from these datasets to recognize patterns, understand context, and generate appropriate responses. The more extensive and diverse the dataset, the better the chatbot becomes at understanding and responding to a wide range of customer queries. Therefore, careful consideration and selection of the datasets used in training are crucial for developing chatbots that deliver high-quality customer service experiences.
• Datasets serve as the foundation for training chatbots to interact intelligently with users
• They contain relevant customer service information such as FAQs and product details
• Utilizing datasets allows developers to create chatbots that provide accurate and timely support
• Datasets enable machine learning algorithms to make connections between user queries and responses
• Training the chatbot with diverse and extensive datasets improves its understanding of customer queries
• Careful consideration and selection of datasets are crucial for developing high-quality chatbot experiences
Key Factors to Consider when Sourcing Customer Service Chatbot Datasets
When sourcing customer service chatbot datasets, there are several key factors to consider in order to ensure the effectiveness and accuracy of the chatbot’s responses. One important factor is the relevance of the dataset to the specific industry or domain of the chatbot. It is crucial to source datasets that are aligned with the products, services, or processes that the chatbot will be trained to assist with. By using industry-specific datasets, the chatbot can better understand and respond to customer inquiries, leading to a more personalized and satisfactory customer experience.
Another factor to consider is the size and diversity of the dataset. A larger dataset can provide the chatbot with a wider range of examples and scenarios to learn from, allowing for more accurate and robust responses. Additionally, including diverse sources of data, such as different customer demographics or languages, can help the chatbot adapt to a wider range of customer needs and preferences.
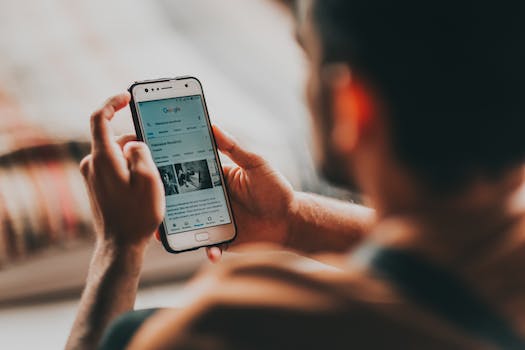
Popular Open-Source Datasets for Training Customer Service Chatbots
There are several popular open-source datasets available that can be used for training customer service chatbots. These datasets contain a wide range of conversations and queries that cover various aspects of customer service interactions. One such dataset is the Cornell Movie Dialogs Corpus, which consists of conversations between characters from over 617 movies. This dataset can be particularly useful for training chatbots to understand and respond to natural language in a conversational manner.
Another popular open-source dataset is the Ubuntu Dialogue Corpus, which contains conversations extracted from the Ubuntu chat logs. This dataset focuses specifically on technical support conversations, making it ideal for training chatbots that will be used in customer service settings related to technology and software. The Ubuntu Dialogue Corpus provides a valuable resource for training chatbots to understand and address specific technical issues that customers may encounter.
Top Commercial Datasets for Training Customer Service Chatbots
Commercial datasets provide a valuable resource for training customer service chatbots to deliver exceptional user experiences. These datasets offer a comprehensive range of real-life scenarios and interactions, enabling chatbots to gain insights into customer behavior and preferences. With a focus on commercial applications, these datasets are carefully curated to reflect the specific needs and challenges of businesses in various industries.
One notable commercial dataset for training customer service chatbots is the “Customer Support on Twitter” dataset, which comprises a vast collection of tweets related to customer support queries.
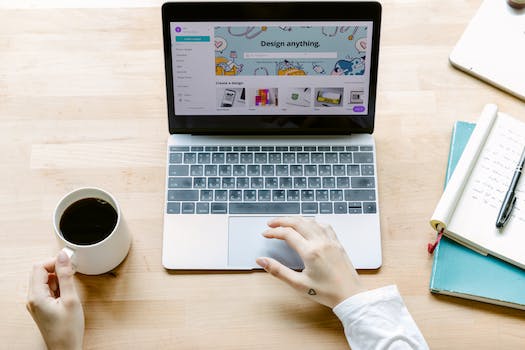
Evaluating the Quality and Relevance of Customer Service Chatbot Datasets
Evaluating the quality and relevance of customer service chatbot datasets is crucial in ensuring the successful training and deployment of chatbot systems. With the abundance of datasets available, it is essential for developers and organizations to carefully assess the suitability of these datasets for their specific chatbot needs.
One key aspect to consider is the source of the dataset. It is important to verify the credibility and reliability of the provider, as this ensures the accuracy and trustworthiness of the data. Additionally, the dataset should be obtained from a relevant domain or industry to ensure its applicability in training customer service chatbots. Using datasets that are specific to the desired chatbot’s field can help improve the bot’s understanding and responses to industry-specific queries. By evaluating the quality and relevance of customer service chatbot datasets, developers can lay the foundation for a robust and effective chatbot system that meets the needs of both the organization and its customers.
Best Practices for Preparing and Annotating Datasets for Chatbot Training
When it comes to preparing and annotating datasets for chatbot training, there are a few key best practices to keep in mind. Firstly, it is crucial to ensure the datasets are representative of the types of conversations the chatbot will be engaging in. This means including a diverse range of topics, conversation styles, and customer queries. By capturing the breadth of possible interactions, the chatbot will be better equipped to handle a variety of customer needs.
In addition to the content of the datasets, it is important to pay attention to the quality of the annotations. Accurate and comprehensive annotations provide the chatbot with the necessary context to understand and respond appropriately to customer queries. This involves properly labeling intents, entities, and other relevant information within the dataset. Additionally, it can be helpful to have multiple annotators review and verify the annotations to ensure consistency and accuracy. By following these best practices for dataset preparation and annotation, developers can set their chatbots up for success in providing effective customer service solutions.
Challenges and Limitations of Using Customer Service Chatbot Datasets
Developing and training customer service chatbots heavily relies on the availability and quality of datasets. However, there are several challenges and limitations that organizations must be aware of when utilizing these datasets. One of the major challenges is the data bias that can be present within the datasets. Since these datasets are often created by humans, they may inadvertently include biases, such as gender or cultural biases, which can affect the chatbot’s responses and interactions with customers. This can lead to inconsistencies in the customer experience and potentially damage the brand’s reputation. It is crucial for organizations to carefully analyze and address any biases within the datasets to ensure fairness and inclusivity in the chatbot’s interactions.
Another limitation of using customer service chatbot datasets is the lack of real-time and dynamic data. Chatbot datasets are typically created based on past customer interactions, which may not reflect the current needs and expectations of customers. As a result, the chatbot may struggle to effectively handle emerging issues or provide timely and relevant solutions. This limitation becomes even more pronounced in industries where customer preferences and trends change rapidly. Organizations need to regularly update and augment their datasets to keep pace with the evolving customer needs and ensure that the chatbot remains effective and valuable in delivering exceptional customer service.
Strategies for Customizing and Augmenting Datasets to Improve Chatbot Performance
When it comes to improving chatbot performance, customizing and augmenting datasets can play a crucial role. One strategy is to incorporate domain-specific data into the training dataset. By including conversations or queries related to specific industries or topics, chatbots can be trained to better understand and respond to industry-specific queries. This can enhance the chatbot’s ability to provide accurate and relevant information to users.
Another strategy is to augment datasets with sentiment analysis. By analyzing the sentiment of user queries or responses, chatbots can better understand the emotions behind the conversation. This can enable chatbots to respond more empathetically and appropriately, enhancing the overall user experience. Augmenting datasets with sentiment analysis can provide valuable insights into customer satisfaction levels and help businesses identify areas for improvement in their chatbot interactions.
Expert Tips for Selecting the Best Customer Service Chatbot Datasets for Training
When it comes to selecting the best datasets for training customer service chatbots, it is crucial to follow a systematic approach. Here are some expert tips to help you navigate through the vast array of options and make an informed decision.
First and foremost, consider the relevance of the dataset to your specific chatbot application. Look for datasets that align with your industry, target audience, and the types of queries your chatbot is expected to handle. This will ensure that the acquired data accurately represents the scenarios your chatbot will encounter in real-world customer interactions.
Secondly, assess the quality of the dataset. Look for datasets that are well-annotated and have a sufficient amount of high-quality data. The annotations should be accurate, consistent, and comprehensive, covering various aspects such as intent classification, entity extraction, and sentiment analysis. Additionally, consider the diversity of the data to ensure your chatbot can handle a wide range of customer queries effectively.
By following these expert tips, you can enhance the training process of your customer service chatbot and equip it with the most suitable datasets. Remember that selecting the right datasets plays a critical role in the success of your chatbot’s performance, so invest time and effort in sourcing the best data available.
Why is data important in training customer service chatbots?
Data plays a crucial role in training customer service chatbots as it helps them learn and understand patterns, improve their responses, and provide accurate and relevant information to customers.
What is the role of datasets in developing effective chatbots?
Datasets provide the necessary information and examples for chatbots to learn from. They help in training chatbots to understand customer queries, identify intents, and generate appropriate responses.
What key factors should be considered when sourcing customer service chatbot datasets?
When sourcing datasets for customer service chatbots, it is important to consider factors such as data quality, relevance to the target domain, diversity of examples, and data privacy and security.
Can you suggest some popular open-source datasets for training customer service chatbots?
Some popular open-source datasets for training customer service chatbots include Cornell Movie Dialogs Corpus, Ubuntu Dialogue Corpus, and Microsoft Dialogue Dataset.
What are some top commercial datasets for training customer service chatbots?
Some top commercial datasets for training customer service chatbots include ChatGPT Dataset, Rasa NLU Training Data, and IBM Watson Conversation Service Datasets.
How can one evaluate the quality and relevance of customer service chatbot datasets?
The quality and relevance of customer service chatbot datasets can be evaluated by assessing factors like accuracy of annotations, coverage of different customer queries, and alignment with the target customer service domain.
What are some best practices for preparing and annotating datasets for chatbot training?
Best practices for preparing and annotating datasets for chatbot training include using clear and consistent annotation guidelines, involving domain experts for accurate labeling, and regularly reviewing and updating the dataset.
What are the challenges and limitations of using customer service chatbot datasets?
Challenges and limitations of using customer service chatbot datasets include bias in the data, lack of diversity in examples, and the need for continuous dataset updates to keep up with evolving customer queries.
How can datasets be customized and augmented to improve chatbot performance?
Datasets can be customized and augmented by adding new examples, incorporating feedback from users, and using techniques like data augmentation and transfer learning to enhance the performance of chatbots.
What are some expert tips for selecting the best customer service chatbot datasets for training?
Some expert tips for selecting the best customer service chatbot datasets for training include considering domain relevance, ensuring data diversity, assessing data quality, and considering the scalability and sustainability of the dataset.